Research progress in deep learning for wavefront reconstruction and wavefront prediction
-
摘要: 深度学习技术与自适应光学技术的结合,预期能够有效提升波前校正效果,并能更好地应对更复杂的环境条件。详细梳理了在波前重构技术和波前预测技术方向上应用深度学习的研究进展,包括研究者在这两个研究方向中所采用的具体研究方法以及相应的神经网络结构设计,同时分析了这些神经网络在不同实际应用场景下的性能表现,并对不同神经网络结构之间的差异进行了比较和讨论,探究了结构差异所带来的具体影响。最后,总结了深度学习在这两个方向上的已有方法,并就未来深度学习与自适应光学技术如何深度融合的发展趋势进行了展望。Abstract: The combination of deep learning technology and adaptive optics technology is expected to effectively improve the wavefront correction effect and better cope with more complex environmental conditions. The research progress of applying deep learning in the direction of wavefront reconstruction and wavefront prediction is detailed, including the specific research methods and corresponding neural network structure design adopted by the researchers in these two research directions, and the performance of these neural networks in different practical application scenarios is analyzed, and the differences between the different neural network structures are compared and discussed, and the specific impacts of the structural differences are explored. The differences between the different neural network structures are compared and discussed, and the specific impacts brought by the structural differences are explored. Finally, the existing methods of deep learning in these two directions are summarized, and the future development trend of the deep integration of deep learning and adaptive optics technology is also prospected.
-
Key words:
- deep learning /
- adaptive optics /
- wavefront reconstruction /
- wavefront prediction
-
表 1 实验中估计的Zernike系数的精度(RMSEs)总结[23]
Table 1. Summary of the accuracies (RMSEs) of the estimated Zernike coefficients in the experiments[23]
Zernike coefficient In-focus Over exposure Defocus Scatter point source 0.142±0.032 0.036±0.013 0.040±0.016 0.057±0.018 extended sources 0.288±0.024 0.214±0.051 0.099±0.064 0.195±0.064 -
[1] 姜文汉. 自适应光学发展综述[J]. 光电工程, 2018, 45:170489Jiang Wenhan. Overview of adaptive optics development[J]. Opto-Electronic Engineering, 2018, 45: 170489 [2] Angel J R P, Wizinowich P, Lloyd-Hart M, et al. Adaptive optics for array telescopes using neural-network techniques[J]. Nature, 1990, 348(6298): 221-224. doi: 10.1038/348221a0 [3] Nemoto K, Fujii T, Goto N, et al. Transformation of a laser beam intensity profile by a deformable mirror[J]. Optics Letters, 1996, 21(3): 168-170. doi: 10.1364/OL.21.000168 [4] Amirabadi M A, Kahaei M H, Nezamalhosseini S A. Deep learning based detection technique for FSO communication systems[J]. Physical Communication, 2020, 43: 101229. doi: 10.1016/j.phycom.2020.101229 [5] Senior A W, Evans R, Jumper J, et al. Improved protein structure prediction using potentials from deep learning[J]. Nature, 2020, 577(7792): 706-710. doi: 10.1038/s41586-019-1923-7 [6] Sandler D G, Barrett T K, Palmer D A, et al. Use of a neural network to control an adaptive optics system for an astronomical telescope[J]. Nature, 1991, 351(6324): 300-302. doi: 10.1038/351300a0 [7] Li Zhaokun, Zhao Xiaohui. BP artificial neural network based wave front correction for sensor-less free space optics communication[J]. Optics Communications, 2017, 385: 219-228. doi: 10.1016/j.optcom.2016.10.037 [8] Jia Peng, Ma Mingyang, Cai Dongmei, et al. Compressive shack–Hartmann wavefront sensor based on deep neural networks[J]. Monthly Notices of the Royal Astronomical Society, 2021, 503(3): 3194-3203. doi: 10.1093/mnras/staa4045 [9] Guo Hong, Korablinova N, Ren Qiushi, et al. Wavefront reconstruction with artificial neural networks[J]. Optics Express, 2006, 14(14): 6456-6462. doi: 10.1364/OE.14.006456 [10] Swanson R, Lamb M, Correia C, et al. Wavefront reconstruction and prediction with convolutional neural networks[C]//Proceedings of SPIE 10703, Adaptive Optics Systems VI. 2018: 107031F. [11] DuBose T B, Gardner D F, Watnik A T. Intensity-enhanced deep network wavefront reconstruction in shack-Hartmann sensors[J]. Optics Letters, 2020, 45(7): 1699-1702. doi: 10.1364/OL.389895 [12] Hu Shuwen, Hu Lejia, Gong Wei, et al. Deep learning based wavefront sensor for complex wavefront detection in adaptive optical microscopes[J]. Frontiers of Information Technology & Electronic Engineering, 2021, 22(10): 1277-1288. [13] Hu Lejia, Hu Shuwen, Gong Wei, et al. Learning-based Shack-Hartmann wavefront sensor for high-order aberration detection[J]. Optics Express, 2019, 27(23): 33504-33517. doi: 10.1364/OE.27.033504 [14] Hu Lejia, Hu Shuwen, Gong Wei, et al. Deep learning assisted Shack-Hartmann wavefront sensor for direct wavefront detection[J]. Optics Letters, 2020, 45(13): 3741-3744. doi: 10.1364/OL.395579 [15] He Yulong, Liu Zhiwei, Ning Yu, et al. Deep learning wavefront sensing method for Shack-Hartmann sensors with sparse sub-apertures[J]. Optics Express, 2021, 29(11): 17669-17682. doi: 10.1364/OE.427261 [16] Guo Youming, Wu Yu, Li Ying, et al. Deep phase retrieval for astronomical Shack–Hartmann wavefront sensors[J]. Monthly Notices of the Royal Astronomical Society, 2022, 510(3): 4347-4354. doi: 10.1093/mnras/stab3690 [17] De Bruijne B, Vdovin G, Soloviev O. Extended scene deep learning wavefront sensing[J]. Journal of the Optical Society of America A, 2022, 39(4): 621-627. doi: 10.1364/JOSAA.443436 [18] Paine S W, Fienup J R. Machine learning for improved image-based wavefront sensing[J]. Optics Letters, 2018, 43(6): 1235-1238. doi: 10.1364/OL.43.001235 [19] Jin Yuncheng, Zhang Yiye, Hu Lejia, et al. Machine learning guided rapid focusing with sensor-less aberration corrections[J]. Optics Express, 2018, 26(23): 30162-30171. doi: 10.1364/OE.26.030162 [20] Jin Yuncheng, Chen Jiajia, Wu Chenxue, et al. Wavefront reconstruction based on deep transfer learning for microscopy[J]. Optics Express, 2020, 28(14): 20738-20747. doi: 10.1364/OE.396321 [21] Tian Qinghua, Lu Chenda, Liu Bo, et al. DNN-based aberration correction in a wavefront sensorless adaptive optics system[J]. Optics Express, 2019, 27(8): 10765-10776. doi: 10.1364/OE.27.010765 [22] Siddik A B, Sandoval S, Voelz D, et al. Deep learning estimation of modified Zernike coefficients and recovery of point spread functions in turbulence[J]. Optics Express, 2023, 31(14): 22903-22913. doi: 10.1364/OE.493229 [23] Nishizaki Y, Valdivia M, Horisaki R, et al. Deep learning wavefront sensing[J]. Optics Express, 2019, 27(1): 240-251. doi: 10.1364/OE.27.000240 [24] Wang Kaiqiang, Zhang Mengmeng, Tang Ju, et al. Deep learning wavefront sensing and aberration correction in atmospheric turbulence[J]. PhotoniX, 2021, 2: 8. doi: 10.1186/s43074-021-00030-4 [25] Ju Guohao, Qi Xin, Ma Hongcai, et al. Feature-based phase retrieval wavefront sensing approach using machine learning[J]. Optics Express, 2018, 26(24): 31767-31783. doi: 10.1364/OE.26.031767 [26] Xin Qi, Ju Guohao, Zhang Chunyue, et al. Object-independent image-based wavefront sensing approach using phase diversity images and deep learning[J]. Optics Express, 2019, 27(18): 26102-23119. doi: 10.1364/OE.27.026102 [27] Ma Huimin, Liu Haiqiu, Qiao Yan, et al. Numerical study of adaptive optics compensation based on convolutional neural networks[J]. Optics Communications, 2019, 433: 283-289. doi: 10.1016/j.optcom.2018.10.036 [28] Guo Hongyang, Xu Yangjie, Li Qing, et al. Improved machine learning approach for wavefront sensing[J]. Sensors, 2019, 19: 3533. doi: 10.3390/s19163533 [29] Wu Yu, Guo Youming, Bao Hua, et al. Sub-millisecond phase retrieval for phase-diversity wavefront sensor[J]. Sensors, 2020, 20: 4877. doi: 10.3390/s20174877 [30] Jorgenson M B, Aitken G J M. Prediction of atmospherically induced wave-front degradations[J]. Optics Letters, 1992, 17(7): 466-468. doi: 10.1364/OL.17.000466 [31] Montera D A, Welsh B M, Roggemann M C, et al. Processing wave-front-sensor slope measurements using artificial neural networks[J]. Applied Optics, 1996, 35(21): 4238-4251. doi: 10.1364/AO.35.004238 [32] McGuire P C, Sandler D G, Lloyd-Hart M, et al. Adaptive optics: neural network wavefront sensing, reconstruction, and prediction[C]//Proceedings of the 194th W. E. Heraeus Seminar. 1999: 97-138. [33] Gallant P J, Aitken G J M. Genetic algorithm design of complexity-controlled time-series predictors[C]//2003 IEEE XIII Workshop on Neural Networks for Signal Processing. 2003: 769-778. [34] 颜召军, 李新阳. 基于神经网络的自适应光学系统变形镜控制电压预测方法[J]. 光学学报, 2010, 30(4):911-916 doi: 10.3788/AOS20103004.0911Yan Zhaojun, Li Xinyang. Neural network prediction algorithm for control voltage of deformable mirror in adaptive optical system[J]. Acta Optica Sinica, 2010, 30(4): 911-916 doi: 10.3788/AOS20103004.0911 [35] 史晓雨, 冯勇, 陈颖, 等. 自适应光学系统变形镜控制电压预测[J]. 强激光与粒子束, 2012, 24(6):1281-1286 doi: 10.3788/HPLPB20122406.1281Shi Xiaoyu, Feng Yong, Chen Ying, et al. Predicting control voltages of deformable mirror in adaptive optical system[J]. High Power Laser and Particle Beams, 2012, 24(6): 1281-1286 doi: 10.3788/HPLPB20122406.1281 [36] 史晓雨, 冯勇, 陈颖, 等. 一种基于并行化方法的自适应光学闭环预测控制器[J]. 光学学报, 2012, 32:0801005 doi: 10.3788/AOS201232.0801005Shi Xiaoyu, Feng Yong, Chen Ying, et al. A novel predictive controller in the adaptive optics control system based on parallelization method[J]. Acta Optica Sinica, 2012, 32: 0801005 doi: 10.3788/AOS201232.0801005 [37] Sun Zhi, Chen Ying, Li Xinyang, et al. A Bayesian regularized artificial neural network for adaptive optics forecasting[J]. Optics Communications, 2017, 382: 519-527. doi: 10.1016/j.optcom.2016.08.035 [38] Wang Ning, Zhu Licheng, Ma Shuai, et al. Deep learning-based prediction algorithm on atmospheric turbulence-induced Wavefront for adaptive optics[J]. IEEE Photonics Journal, 2022, 14(5): 1-10. [39] Chen Ying. Voltages prediction algorithm based on LSTM recurrent neural network[J]. Optik, 2020, 220: 164869. doi: 10.1016/j.ijleo.2020.164869 [40] Chen Ying. LSTM recurrent neural network prediction algorithm based on Zernike modal coefficients[J]. Optik, 2020, 203: 163796. doi: 10.1016/j.ijleo.2019.163796 [41] Liu Xuewen, Morris T, Saunter C, et al. Wavefront prediction using artificial neural networks for open-loop adaptive optics[J]. Monthly Notices of the Royal Astronomical Society, 2020, 496(1): 456-464. doi: 10.1093/mnras/staa1558 [42] Wu Ji, Tang Ju, Zhang Mengmeng, et al. PredictionNet: a long short-term memory-based attention network for atmospheric turbulence prediction in adaptive optics[J]. Applied Optics, 2022, 61(13): 3687-3694. doi: 10.1364/AO.453929 [43] Swanson R, Lamb M, Correia C M, et al. Closed loop predictive control of adaptive optics systems with convolutional neural networks[J]. Monthly Notices of the Royal Astronomical Society, 2021, 503(2): 2944-2954. doi: 10.1093/mnras/stab632 -
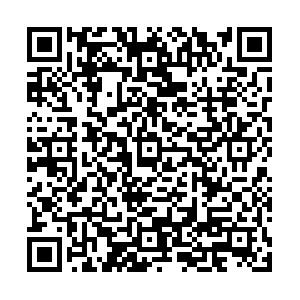
计量
- 文章访问数: 44
- HTML全文浏览量: 19
- PDF下载量: 8
- 被引次数: 0