Research of aircraft pose estimation based on neural network feature line extraction
-
摘要: 为实现复杂情况下的飞机位姿识别,提出了基于神经网络特征线提取的位姿识别新方法。该方法利用3D模型进行图像渲染,通过添加背景形成数据集,为提高算法鲁棒性进行了数据集增强。特征线提取模型采用卷积神经网络提取目标深度特征,利用热力图获取飞机特征线。结合飞机特征线、飞机3D模型以及n线透视方法解算目标位姿。该方法建立的飞机特征线提取模型,在复杂背景下准确率约为91%。叠加了各类噪声后,准确率为84%。飞机位姿通过EPnL算法与非线性优化进行求解。在目标背景复杂的情况下,实验得到的平均预测角度误差约为0.57°,平均预测平移误差约为0.47%。图像叠加各类噪声后,得到的平均预测角度误差约为2.11°,平均预测平移误差约为0.93%。本文提出的飞机位姿识别方法在复杂背景、各类噪声影响下可以较精准的预测飞机位姿,应用场景更加广泛。Abstract: In order to estimate the aircraft pose in complex situation, this paper proposes a new method of aircraft pose estimation based on neural network line extraction. This method uses 3D model to render images, and forms dataset through adding backgrounds. The dataset is enhanced to make the algorithm robust. The line extraction model uses convolutional neural network to extract deep features, and uses heatmap to obtain aircraft feature lines. The target pose is solved by combining the aircraft feature line, the aircraft 3D model and the perspective-n-line method. The accuracy of the line extraction model is 91% in complex background. The accuracy is 84% after adding sorts of noises. The aircraft pose is solved by using EPnL algorithm and nonlinear optimization. The average angle error is about 0.57°, and the average translation error is about 0.47% when the target is in a complex background. After adding sorts of noises to the image, the average angle error is about 2.11°, and the average translation error is about 0.93%. The aircraft pose estimation method proposed in this article can accurately predict the aircraft pose under complex backgrounds and various types of noise, and its application scenarios are more extensive.
-
Key words:
- neural network /
- line extraction /
- pose estimation /
- dataset enhancement
-
表 1 全连接检测头测试结果
Table 1. results of fully connected head
No. output feature precision/% 1 $ k,b $ 36.39 2 $ k,d $ 71.35 3 $ \theta ,b $ 18.57 4 $ \theta ,d $ 73.15 5 $ \theta ,x,y $ 76.56 表 2 不同骨架测试准确率
Table 2. precision of different backbones
表 3 不同情况下的平均预测误差
Table 3. Average error of pose estimation in different situations
situation 7 lines model error/% 17 lines model error/% pure background 1.04 1(.24°) 0.52 (1.53°) complex background 1.05 (1.21°) 0.47 (0.57°) complex background and noises 3.49 (4.61°) 0.93 (2.11°) -
[1] 刘刚, 梁晓庚, 张京国. 基于红外图像的飞机目标关键攻击部位识别[J]. 计算机工程与应用, 2011, 47(24):174-177,228Liu Gang, Liang Xiaogeng, Zhang Jingguo. Recognizing airplane’s key attacking part based on infrared image[J]. Computer Engineering and Applications, 2011, 47(24): 174-177,228 [2] 成杰, 李新德. 基于Hu矩与改进PNN的飞机姿态识别算法[J]. 航空兵器, 2017(1):55-61Cheng Jie, Li Xinde. An algorithm of aircraft pose recognition based on Hu moments and IPNN[J]. Aero Weaponry, 2017(1): 55-61 [3] 王宏波, 庄志洪, 张清泰. 基于动态红外图像的飞机姿态识别方法研究[J]. 红外与激光工程, 2003, 32(1):8-12Wang Hongbo, Zhuang Zhihong, Zhang Qingtai. Study on recognition of aircraft status based on dynamic Infrared images[J]. Infrared and Laser Engineering, 2003, 32(1): 8-12 [4] 王宏波, 庄志洪, 张清泰. 一种红外成像引信中的飞机姿态识别方法[J]. 宇航学报, 2003, 24(4):354-358 doi: 10.3321/j.issn:1000-1328.2003.04.006Wang Hongbo, Zhuang Zhihong, Zhang Qingtai. A recognizing method of aircraft status in imaging infrared fuze[J]. Journal of Astronautics, 2003, 24(4): 354-358 doi: 10.3321/j.issn:1000-1328.2003.04.006 [5] 冀芳, 武瑞娟, 何红丽. 大型外挂物投放影像测量亚像素定位技术[J]. 计算机与数字工程, 2017, 45(8):1647-1651Ji Fang, Wu Ruijuan, He Hongli. Sub pixel positioning technology for large external store image measurement[J]. Computer and Digital Engineering, 2017, 45(8): 1647-1651 [6] Santos N P, Melício F, Lobo V, et al. A ground-based vision system for UAV pose estimation[J]. International Journal of Robotics and Mechatronics, 2014, 1(4): 138-144. [7] Su Guozhong, Zhang Jianqing, Zheng Shunyi. Moving objects pose acquisition from image sequence[C]//Proceedings of the 20th ISPRS Congress Technical Commission V. 2004: 730-734. [8] 李兴唐, 郭立红. 基于三维模型的飞机识别与姿态估计[J]. 武汉理工大学学报, 2008, 30(8):74-77Li Xingtang, Guo Lihong. Aircraft recognition and pose estimation base on 3D model[J]. Journal of Wuhan University of Technology, 2008, 30(8): 74-77 [9] 王习文, 马军, 陈娟, 等. 飞机三维姿态测量的角平分线方向向量法[J]. 光学 精密工程, 2010, 18(2):369-376Wang Xiwen, Ma Jun, Chen Juan, et al. Direction vector method of angle bisector lines for measuring aircraft’s 3D attitude[J]. Optics and Precision Engineering, 2010, 18(2): 369-376 [10] 王彬, 何昕, 魏仲慧. 采用单目图像特征直线的飞机姿态估计[J]. 计算机测量与控制, 2013, 21(2):473-476 doi: 10.3969/j.issn.1671-4598.2013.02.061Wang Bin, He Xin, Wei Zhonghui. A method of measuring pose of aircraft from mono-view based on line features[J]. Computer Measurement & Control, 2013, 21(2): 473-476 doi: 10.3969/j.issn.1671-4598.2013.02.061 [11] 王彬, 何昕, 魏仲慧. 采用多站图像直线特征的飞机姿态估计[J]. 光学 精密工程, 2013, 21(7):1831-1839 doi: 10.3788/OPE.20132107.1831Wang Bin, He Xin, Wei Zhonghui. Attitude estimation of aircrafts using line features on multi-camera images[J]. Optics and Precision Engineering, 2013, 21(7): 1831-1839 doi: 10.3788/OPE.20132107.1831 [12] Teng Xichao, Yu Qifeng, Luo Jing, et al. 2019. Aircraft pose estimation based on geometry structure features and line correspondences[J]. Sensors, 2019, 19: 2165. doi: 10.3390/s19092165 [13] Li Yibo, Yu Ruixing, Zhu Bing. 2D-key-points-localization-driven 3D aircraft pose estimation[J]. IEEE Access, 2020, 8: 181293-181301. doi: 10.1109/ACCESS.2020.3026209 [14] Fan Runze, Xu Tingbing, Wei Zhenzhong. Estimating 6D aircraft pose from keypoints and structures[J]. Remote Sensing, 2021, 13: 663. doi: 10.3390/rs13040663 [15] 王平, 何卫隆, 张爱华, 等. EPnL: 一种高效且精确的PnL问题求解算法[J]. 自动化学报, 2022, 48(10):2600-2610Wang Ping, He Weilong, Zhang Aihua, et al. EPnL: an efficient and accurate algorithm to the PnL problem[J]. Acta Automatica Sinica, 2022, 48(10): 2600-2610 [16] He Kaiming, Zhang Xiangyu, Ren Shaoqing, et al. Deep residual learning for image recognition[C]//Proceedings of 2016 IEEE Conference on Computer Vision and Pattern Recognition. 2016: 770-778. [17] Xie Saining, Girshick R, Dollár R, et al. Aggregated residual transformations for deep neural networks[C]//Proceedings of 2017 IEEE Conference on Computer Vision and Pattern Recognition. 2017: 5987-5995. [18] Sun Ke, Xiao Bin, Liu Dong, et al. Deep high-resolution representation learning for human pose estimation[C]//Proceedings of 2019 IEEE/CVF Conference on Computer Vision and Pattern Recognition. 2019: 5686-5696. [19] Huang Gao, Liu Zhuang, Van Der Maaten L. Densely connected convolutional networks[C]//Proceedings of 2017 IEEE Conference on Computer Vision and Pattern Recognition. 2017: 2261-2269. [20] Sandler M, Howard A, Zhu Menglong, et al. MobileNetV2: inverted residuals and linear bottlenecks[C]//Proceedings of 2018 IEEE/CVF Conference on Computer Vision and Pattern Recognition. 2018: 4510-4520. -
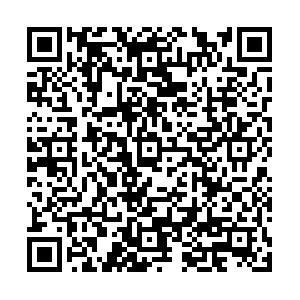
计量
- 文章访问数: 41
- HTML全文浏览量: 24
- PDF下载量: 7
- 被引次数: 0