Infrared image segmentation based on PCNN with genetic algorithm parameter optimization
-
摘要: 构造一种基于遗传算法参数优化的脉冲耦合神经网络(PCNN)红外图像分割算法。该算法首先利用PCNN的全局耦合性和脉冲同步性对输入图像进行点火处理,根据PCNN的输出结果计算熵作为遗传算法的适应度函数,并利用熵的变化量作为遗传算法的收敛依据,对PCNN模型中影响图像分割的参数进行组合优化,结合PCNN生物视觉特性和遗传算法解空间随机搜索能力来寻找关键参数的最优值。将遗传算法和PCNN进行结合可充分发挥二者优势,将本文方法与最大类间方差法(OTSU)、最大熵直方图分割算法和PCNN分割方法进行对比,通过交叉熵、区域对比度等客观指标对分割后的图像进行定量分析,结果表明无论从主观视觉还是客观指标,本文方法分割效果优于其他对比方法。Abstract: A PCNN infrared image segmentation algorithm based on genetic algorithm parameter optimization is proposed. The algorithm first carries out the ignition process for input images using PCNN global coupling and pulse synchronization. Entropy is calculated according to the PCNN output and used for the fitness function of genetic algorithm. The entropy change is used as the convergence criterion of genetic algorithm. Combination of optimization is made for parameters effecting image segmentation in PCNN model. To find the optimal values of key parameters biological visual characteristics of PCNN and solution space random search of the genetic algorithm are adopted. Combination of genetic algorithm and PCNN can make full use of the advantages. Compared with OTSU, maximum entropy histogram segmentation algorithm and PCNN segmentation method, quantitative analysis is conducted for the image after segmentation using cross entropy and region contrast objective index. Simulation results show that, judging either by subjective vision or by objective index, the proposed method is superior to other comparative method in segmentation effect.
-
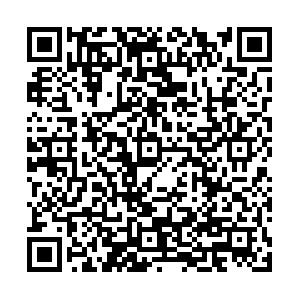
计量
- 文章访问数: 2226
- HTML全文浏览量: 367
- PDF下载量: 426
- 被引次数: 0